The AI Paradox for VC
The launch of ChatGPT has sparked a wave of excitement around AI’s potential. In just two months, it has grown to 100M users globally, making it the fastest growing consumer product in history. It’s captured the imagination of everyday consumers and businesses alike on creative ways to leverage LLMs. Venture capital firms are no different. VC firms are rapidly embracing AI to augment their investment processes to drive efficiency and generate alpha.
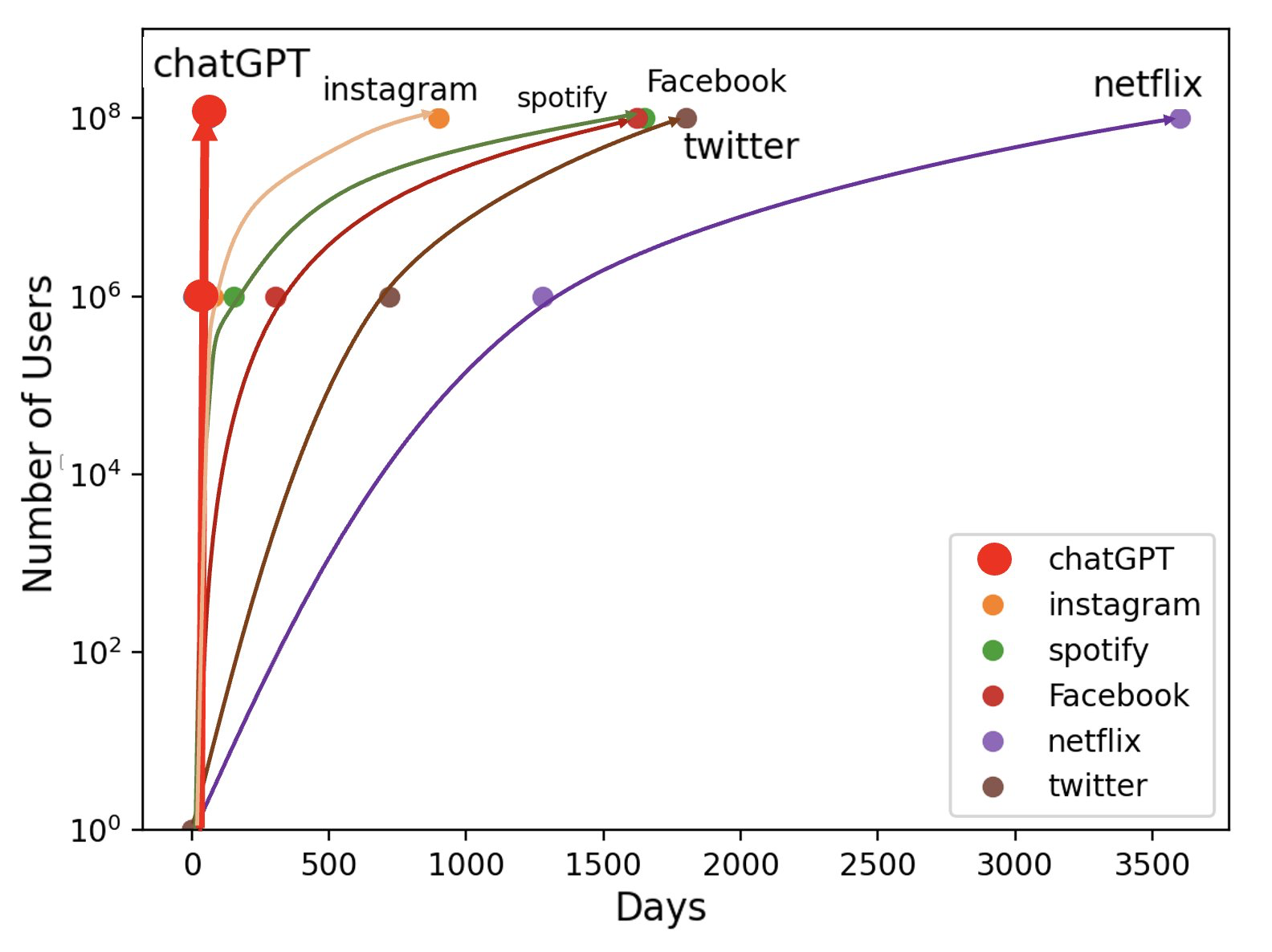
In this post, I explore how AI is likely to have a paradoxical impact on the venture capital asset class. While it will likely be instrumental in improving venture investment processes and drive efficiency, it will simultaneously erode alpha.
While we are still in the early innings of understanding full-scale AI capabilities, let’s explore how AI augmentation improves the venture process.
AI in Venture Capital
- Data Ingestion and Synthesis
Venture success depends on data - consumer and enterprise behavior, speed and scale of technological innovation adoption, and historical performance and outcomes. Past and present data forms the backbone of venture pattern matching. The synthesis of this data informs each step in the VC process, from sourcing, to diligence, and post investment support. Naturally, this process is slow and time consuming and can take weeks or months at some firms. AI augmentation can drastically improve this process. Firms are already using AI tools like ChatGPT (General), BloombergGPT and Finchat (Financial Data), Bardeen (Sourcing), Otter (Note Taking), RoseAI (Data Transformation), Numerous (Data Analysis), and Gamma (Deck generation) to improve efficiency, accuracy, and quality of their process while reducing cycle time. In part, this has the potential to help VCs overcome vulnerabilities in the venture process: incomplete data and confirmation bias. As a result, decision making becomes better, faster, and more “complete”. - Automation
The cost equation in a venture capital business is that of a services business. Human capital intensive, manual process of sourcing, diligence, and portfolio management. VCs have historically embraced technology and data to incrementally improve operating leverage such as Pitchbook licenses for sourcing, fund management tools like AngelList, and cap table management like Carta. Yet the vast majority of processes are labor intensive and constrained by the number of employees (which itself is constrained by management fee), and time. In the last two months, we have seen a glimpse of the possibilities of autonomous automation with tools like AutoGPT and BabyGPT. These tools redefine business process automation. Instead of needing fully repeatable steps with painstaking documentation, they create a self-improving autonomous agent that defines its own scope of work, and iterates on its own process to get to the result. While not quite ready for primetime as of this writing, this creates unique possibilities to deploy nearly infinite VC analysts at scale conducting research 24x7 in the background. Over time, a firm's institutional knowledge will rapidly accumulate based on output of these agents, reduce cycle time during diligence, and expand the firm’s aperture and throughput without an increase in manual labor. As marginal cost of deploying these agents go down, and quality of iteration and output increase, the VC business will start to see a drastic improvement in operational leverage. Plus it will get firms more shots at goal.
This combination of data ingestion and synthesis, and automation has the potential to (a) enable firms to (near) infinitely increase deal processing bandwidth, increasing the aperture for success (and luck), and (b) lower the cost of scaling investment teams to deploy larger amounts of money.
AI Paradox: Alpha Erosion
However, increased efficiency and throughput are not sufficient conditions for better returns in this asset class. VC is competitive. It only became more competitive In a zero interest rate policy environment with angels, super angels, syndicates, rolling funds, sector specific funds, stage specific funds, multi-stage funds, family offices, and even non-traditional funds from halfway across the world all competing for deals.
Success in VC essentially boils down to risk asymmetry in inefficient private markets. Through complete information, zero marginal cost access, and low cost infinite parallel synthesis, AI can level the playing field making private markets more symmetrical and efficient. This would force firms to flex up on pricing in competitive rounds, and increase investing in their brand and positioning.
Let’s explore how AI’s advantages turn into alpha eroders for VCs.
“Perfect” Information & Homogenous Decision Making
VC alpha comes from making contrarian bets driven by unique insights on a market or founder. Investing in the dotted line future trend of a technology or emergent consumer behavior that no one else has seen or synthesized the impact of. Now let’s imagine a world where AI, with all its advantages above, has deeply penetrated the VC process. Foundational models are trained on similar historical data. As a result, these models will quickly show a propensity to identify the same opportunities and analyze markets in similar ways. Contrarianism goes out the window. More venture funds start chasing the same deals. This crowding leads to higher entry prices and as long as the scale of outcomes does not change by an order of magnitude, lead to depressed returns across the asset class as a whole.
It will also lower the variance between firms. Below median firms can quickly climb the adoption curve to match data ingestion, analysis, and synthesis levels of the top firms and identify similar opportunities quickly. Now, all they have to do is throw their hat in the ring and try to win on brand or price. Every founder has a slightly different brand-price elasticity curve, so in aggregate, these firms would (a) drive up price in deals they lose, lowering other firms’ gross returns, or (b) win some deals with good outcomes they would not have previously gotten into, but at a higher price due to competition. As a result, the range of outcomes more tightly clusters around the mean.
AI: Sixth Dimension of Competition
As LLMs rapidly lead to the commodification of public data and squeeze alpha generation as described above, firms will rapidly rush to their own proprietary data sets. Even if all competitors have an identical underlying data set to make decisions, the decision making and prioritization (“weights”) will be influenced by their individual internal proprietary data set. To do this, firms will develop in-house AI expertise to build proprietary tooling on top of foundational models, and competition in venture will shift to the success of each firm’s in-house model. So, VC investment teams will have to evolve as well. Instead of researchers and analysts, firms will deploy multiple instances of autonomous agents for sourcing and diligence, and supplement with prompt engineers to sharpen the outputs from their proprietary models. Where historically firms competed in the five dimensional space of information asymmetry, access, price, brand, and speed, the asset class will evolve to compete on the three dimensions of AI productivity, brand, and price. Firms like SignalFire already have AI as a core part of their DNA. Firms like 776 are integrating and experimenting. Spark Capital is hiring for AI "tinkerers". The race has begun.
Consequently, I expect the venture asset class to go through a rapid metamorphosis and emerge with four new characteristics:
Lower returns as a whole due to increased competition
Reduced variance in returns across funds within venture due to an upward shift in competency and efficiency
Competing on specialized AI capability through proprietary models
Multi-stage firms being forced to go even earlier to generate “pre-data” alpha
Venture firms are at a unique inflection point in their evolution. Embracing AI for efficiency, leverage, and throughput will elevate the venture process. However, firms will have to develop new competencies in prompt engineering and AI model creation and optimization. The business model will evolve with more operating leverage enabling more investment in brand and marketing. It might lead to lower management fees to be more competitive for LPs if returns based differentiation isnt enough. Firms will evolve to look like software companies than services firms. While adoption is still early, rapid AI advancements and swift adoption from some VC firms will paint a clearer picture in the next 12-18 months.